
June 28, 2023
Above: Doctoral student Zineng Tang discusses the highlighted research paper “Unifying Vision, Text, and Layout for Universal Document Processing” with a crowd of onlookers during a poster session at CVPR 2023.
The 2023 IEEE/CVF Conference on Computer Vision and Pattern Recognition (CVPR) was held in Vancouver from June 18 to 22. Widely recognized as the premier event in the field of computer vision and pattern recognition, it drew top researchers from all over the world. UNC Computer Science research groups led by faculty members Mohit Bansal, Gedas Bertasius, and Marc Niethammer presented several papers in the conference and also contributed to workshops.
Bansal is a John R. & Louise S. Parker professor of computer science and an expert in multimodal, generative, and explainable artificial intelligence (AI). He delivered a workshop keynote at a workshop titled “Explainable AI for Computer Vision” and served in panel discussions at two other workshops, titled “Open-Domain Reasoning Under Multi-Modal Settings” and “Knowledge-Driven Vision-Language Encoding.” His talk discussed interpretable visual programming frameworks for text-to-image generation and evaluation, then looked at how to supervise model explanations in a right-for-the-right-reason way.
Bertasius, an assistant professor and expert in video understanding, multimodal deep learning, and first-person vision, delivered two keynote talks at CVPR workshops this year: “Long-form Video Understanding and Generation” and “Sight and Sound.” His keynote talks focused on developing efficient and scalable audiovisual video models and evaluating models for long video understanding.
Bansal, Bertasius, and doctoral students Jaemin Cho, Yan-Bo Lin, Yi-Lin Sung, Md Mohaiminul Islam, and Feng Cheng played a significant role in not only presenting research, but also organizing one of the most popular workshops at CVPR. Titled “Transformers for Vision,” the workshop discussed the utility of transformers, a deep learning tool commonly used in natural language processing tasks like language translation, when adapted to computer vision tasks. The workshop attracted an impressive gathering of nearly 500 in-person and online attendees.

Niethammer, a professor and expert in medical image analysis, heads the UNC Biomedical Image Analysis Group (UNC-biag). His team contributed a collaborative paper on medical image registration using deep learning techniques.
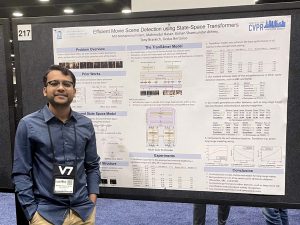
Even though computer vision isn’t the main research field of any of the three research groups, they contributed several papers to the conference, which had an acceptance rate of only 25.8 percent. Those papers focused on improving the way computers analyze video footage and make decisions or complete related tasks based on the content, as well as unified document understanding, visual navigational planning, and image registration. Here, we have summarized some of the research presented at the conference.
HIGHLIGHT: “Unifying Vision, Text, and Layout for Universal Document Processing”
Authors: Zineng Tang (UNC-Chapel Hill), Ziyi Yang (Microsoft), Guoxin Wang (Microsoft), Yuwei Fang (Microsoft), Yang Liu (Microsoft), Chenguang Zhu (Microsoft), Michael Zeng (Microsoft), Cha Zhang (Microsoft), Mohit Bansal (UNC-Chapel Hill)
Selected by the conference as a “Highlight,” which was reserved for only 10 percent of accepted papers and 2.6 percent of submitted papers, this collaboration with researchers from Microsoft produced Universal Document Processing (UDOP), a Document AI model that can handle a variety of task formats involving a combination of text, image, and layout modalities. This is the first time in the field of document AI that one model simultaneously achieves high-quality neural document editing and content customization, which has the potential to change the way we deal with things like finance reports, academic papers, and websites.
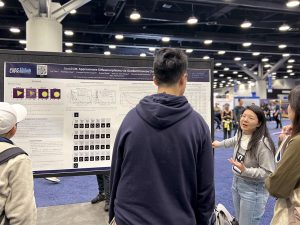
“Efficient Movie Scene Detection Using State-Space Transformers”
Authors: Md Mohaiminul Islam (UNC-Chapel Hill), Mahmudul Hasan (Comcast), Kishan Shamsundar Athrey (Comcast), Tony Braskich (Comcast), Gedas Bertasius (UNC-Chapel Hill)
This paper, in collaboration with Comcast Cable Communications, LLC, improves on existing machine detection of scenes in movies. Computer vision models that can “watch” movies, accurately detecting people and objects and inferring storylines, offer great value to cable television networks and providers and streaming platforms that seek to categorize and recommend content based on thematic elements. Unfortunately, most video detection models are designed for short video segments and struggle to reason over the long video durations required for detection in movies. Working with Comcast, Bertasius and his team developed TranS4mer, a long-range movie scene detection model that outperforms existing algorithms while working faster and greatly decreasing the amount of memory required.
“GradICON: Approximate Diffeomorphisms via Gradient Inverse Consistency”
Authors: Lin Tian (UNC-Chapel Hill), Hastings Greer (UNC-Chapel Hill), François-Xavier Vialard (UGE Noisy-Champs, Paris), Roland Kwitt (PLUS, Salzburg), Raúl San José Estépar (Harvard), Richard Jarrett Rushmore (Boston), Nikolaos Makris (Harvard), Sylvain Bouix (UQAM, Montreal), Marc Niethammer (UNC-Chapel Hill)
“Hierarchical Video-Moment Retrieval and Step-Captioning”
Authors: Abhay Zala* (UNC-Chapel Hill), Jaemin Cho* (UNC-Chapel Hill), Satwik Kottur (Meta), Xilun Chen (Meta), Barlas Oguz (Meta), Yashar Mehdad (Meta), Mohit Bansal (UNC-Chapel Hill)
Working alongside researchers from Meta, Bansal’s team presented a new dataset called HiREST and a joint model for taking a set of thousands of instructional videos and systematically breaking them down into individual clips with textual annotations via a four-step protocol. First, the model retrieves a specific video file based on an input query. Second, the model selects the appropriate moment, or clip, from that video. Next, the model segments the moment into smaller clips, like chapters. Finally, the model generates captions for each segment. The final result is the relevant clip, broken into captioned segments. This dataset and joint model can provide a basis for future research into a very useful computer vision and natural language generation process.
* equal contribution
“Improving Vision-and-Language Navigation by Generating Future-View Image Semantics”
Authors: Jialu Li (UNC-Chapel Hill), Mohit Bansal (UNC-Chapel Hill)
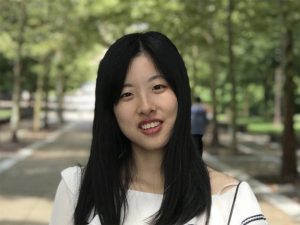
By applying computer vision and natural language processing to robotics, we can enable safer search-and-rescue operations by sending robots into dangerous areas like damaged buildings and have a human guide them with natural language instructions, such as “turn toward the window” or “move forward to the next room”. This process of having a machine agent navigate based on natural language instructions is called vision-and-language navigation, or VLN. While navigating, humans intuitively generate predictions of what a future view may look like, imagining, for example, the layout of the next room once they turn a corner. Bansal and doctoral student Jialu Li worked to improve a machine’s ability to generate a potential future view, thus enhancing its ability to navigate based on human cues.
“VindLU: A Recipe for Effective Video-and-Language Pretraining”
Authors: Feng Cheng (UNC-Chapel Hill), Xizi Wang (IU Bloomington), Jie Lei (Meta, previously UNC-Chapel Hill), David Crandall (IU Bloomington), Mohit Bansal (UNC-Chapel Hill), Gedas Bertasius (UNC-Chapel Hill)
Given text input from a human user, computers can process video to answer questions about the video (video question answering) or retrieve videos from a dataset that match an input query (text-to-video retrieval). As humans generate hours of video footage every second, automating video analysis tasks like these becomes increasingly important. These tasks are classified as video-and-language (VidL) understanding, and most modern approaches use specialized architectures and pretraining protocols which can make the individual models difficult to analyze and compare. Alongside researchers from Indiana University Bloomington, UNC CS researchers conducted a study demystifying the most important factors in the design of these VidL models and developed a step-by-step process for effective VidL pretraining. The process, named “VindLU,” outperforms leading models in text-to-video retrieval and video question answering on 9 distinct video-language benchmarks.
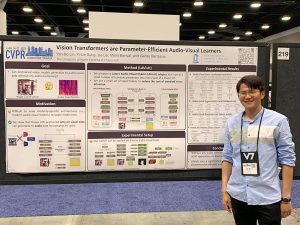
“Vision Transformers Are Parameter-Efficient Audio-Visual Learners”
Authors: Yan-Bo Lin (UNC-Chapel Hill), Yi-Lin Sung (UNC-Chapel Hill), Jie Lei (Meta, previously UNC-Chapel Hill), Mohit Bansal (UNC-Chapel Hill), Gedas Bertasius (UNC-Chapel Hill)
Transformers are a type of deep learning model that are seeing increased usage in computer vision. Known as vision transformers or ViTs when adapted to computer vision, they can be effective in image classification. Research teams led by Bansal and Bertasius determined that ViTs pretrained only on visual data could generalize to audio data for complex audio-visual understanding tasks. Additionally, the team developed an adapter process, called LAVISH, that outperforms existing video- or audio-specific methods while requiring less tuning of parameters initially. Their LAVISH adapter was able to effectively perform audio-visual tasks like pinpointing moments in video (event localization), pinpointing the source of sound in a particular frame (segmentation), and answering natural language questions about the video (question-answering).