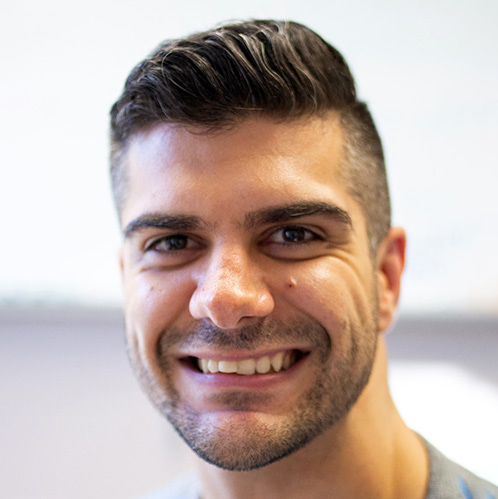
October 17, 2023
Assistant Professor Junier Oliva received a two-year grant from the National Science Foundation to improve the ability of machine learning models to extrapolate beyond the scope of their training dataset. The project will hopefully enhance scientific tasks across numerous disciplines, including chemical discovery and safety assessment.
The grant includes collaborator Alexander Tropsha, who is a professor in the UNC Eshelman School of Pharmacy with an adjunct appointment in the Department of Computer Science.
Machine learning (ML) has the potential to revolutionize many high-impact scientific fields, including chemistry and biology, where models can be used to predict the results of costly processes in order to better guide research strategy and resource allocation. Unfortunately, it is still relatively uncommon for these predictions to be successfully validated by experimentation, because these ML models often struggle with extrapolation, which is the characterization of data that lies outside of the distribution of their training sets. This weakness can be hampering in a field like chemistry, where it can be time-consuming and costly to synthesize and test substances after a false positive. In general, inaccurate or unreliable extrapolation makes it difficult to expand beyond existing scientific knowledge in any field.
Oliva is developing methodologies to improve ML models’ extrapolatory capabilities. His project starts by developing trials to assess how well a model is able to extrapolate beyond the scope of its training set. These assessments can be used to explore the input space of the model in order to anticipate and filter out predictions that are likely to be unreliable. Oliva is also building methodology to guide the acquisition of new training data, letting the researcher know what missing input data could improve the model’s ability to extrapolate.
The project is tackling core problems in both supervised and unsupervised machine learning, statistics, and artificial intelligence. Oliva hopes that it will have important implications for cheminformatics and bioinformatics, with applications in tasks like drug discovery, where molecular features can be correlated with target properties, and ML models can be helpful in discovering new molecules with these properties. He also sees potential applications in autonomous vehicles, public policy, and health care.
Oliva is an assistant professor in the Department of Computer Science, as well as lead faculty in the master of applied data science program, teaching machine learning for the School of Data Science and Society. He leads the LUPA Lab, a research group devoted to machine learning and artificial intelligence. Using techniques ranging from modern deep learning architectures to nonparametric statistics, his research makes strides in areas including high-dimensional density estimation and modeling, sequential modeling and recurrent neural networks, and learning over complex or structured data.